Big COPData Study:
to predict hospitalizations in COPD patients
Fill the form and join the study
Study funded by EC - H2020 SME Instrument, conducted in UK, France, Germany, Switzerland, Belgium, Canada and USA.
Feb 2019 to Oct 2021
-
We aim to identify those factors that are potentially associated with hospital admissions in patients with COPD across Europe, Canada and USA in order to develop a risk prediction model for hospitalization.
-
This is an observational, descriptive study, using data captured from Electronic Health Records (EHRs). Time span of data is the last 5 years of clinical practice included in the EHRs.
In this study we propose to take advantage of a software application (i.e. SAVANA), created in the context of the era of electronic health, to be able to reuse the information included in EHRs. This software application is a powerful natural language processing (NLP) free-text analysis engine, capable of meaningfully interpreting the contents of the EHRs, regardless of the management system in which it operates. In this context, this machine learning analytical method can be used to build a flexible, customized and automated predictive model using the information available in EHRs.
Responsible Parties
- Dr. Ignacio H. Medrano - Chief Medical Officer Medsavana SL
- Jorge Tello - Chief Executive Officer Medsavana SL
- Ana López Ballesteros - RWE Generation Specialist Medsavana SL
Scientific Committee:
- Dr. Julio Ancochea - Hospital Universitario de La Princesa
- Dr. Alberto Fernández - Hospital Universitario de Vigo
- Dr. Borja Cosio - Hospital Universitario Son Espases
- Dr. José Luis Izquierdo - Hospital Universitario de Guadalajara
- Dr. José Luis López Campos - Hospital Universitario Virgen del Rocío
- Dr. Marc Miravitlles - Hospital Universitario Vall d’Hebron
- Dr. José Miguel Rodríguez - Hospital Universitario de Alcalá
- Dr. Juan José Soler-Cataluña - Hospital Universitario Arnau de Vilanova
- Dr. Joan.B. Soriano - Hospital Universitario de La Princesa
The Scientific Committee will be completed with one member from each participating country.
PARTICIPATE IN THE STUDY
Predicting hospitalizations in COPD patients across Europe
through Big Data, Artificial Intelligence (AI) and Natural Language Processing
Big Data in healthcare
Big Data defines a new method of generating knowledge that is possible due to two complimentary phenomena:
- Exponential accumulation of data, thanks to network collaboration (internet)
- Growing computing capacity to process it.
Big Data implies data reuse across different large volume databases with exploration purposes, other than those for which they were originally structured and populated.
Big Data is an extension of Statistics, as it virtually manages “the entire” set of events related to the studied fact, so that it interrelates a large enough number of variables, in order to infer correlations where the human mind is not able to do so.
Medical information is estimated to double every 5 years.
«Probably, no human activity generates as much data as healthcare»
AI in healthcare
AI describes the technology that allows computers to perform operations originally attributed uniquely to humans, such as pattern recognition, language, prediction…
The process by which machines are able to learn after having seen numerous examples of the same element is known as «machine learning».
This computational task has become exponentially more efficient thanks to the imitation of human neural networks, in what is called «deep learning».
The main applications of artificial intelligence in healthcare are:
- Medical natural language processing, which allows to exploit free or unstructured text from electronic health records.
- Identification of diagnostic or therapeutic patterns, which resemble the common practice of physicians but derived from insights gained from the collective data sets.
- Predictive analysis, which enables the anticipation of clinical events from patient clusters thereby stratifying their risk.
Electronic Medical Records (EMR) Reuse
The data recorded by clinicians during their usual practice generates a huge amount of valuable information. This is the representation of what happens with the attended casuistry in the real world, under the uncertain conditions of the environment.
A fundamental requirement in order to accelerate and expand the extraction process is the implementation of electronic health records systems, which not only allow information sharing (interoperability among levels of care) but also the reuse of it.
We can divide the reuse of the EMR into images, numbers and text:
- Images: more easily exploitable by an artificial intelligence and already existing cases today that overcome the predictive and diagnostic capacity of humans (the case of Google Deepmind diagnosing diabetic retinopathy, melanoma, breast cancer, pneumonia on chest x-ray…)
- Numbers: also, existing success cases today (prediction in ICU, prediction of hospital mortality, readmissions, stays…)
- Text
Medical Natural Language Processing
It is just not possible for the clinician to structure or codify every concept recorded in health records.
The granularity of information cannot be achieved unless free text interpretation techniques are used, which can capture all the richness of them. These techniques are grouped under one of the branches of artificial intelligence, called «Natural Language Processing».
It implies the combination of several techniques, in order to detect concepts and relationships among them. Relying on linguistic tools, statistics, databases and medical knowledge, this technology is able to disambiguate the particularities of human language: spelling mistakes, different ways of expressing negatives or speculations, use of acronyms, subjectivity, anaphora, subordinates … to determine which univocal concepts correspond to each clinical annotation.
Request more information
Do you want to know more?
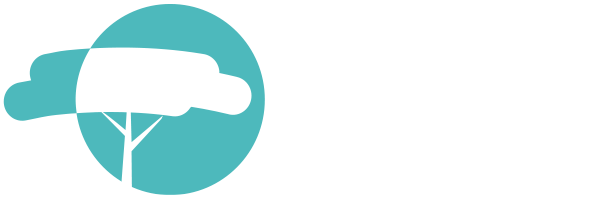
AI + RWE
Savanamed.com © 2019 | Legal notice | Privacy policy | Cookies Policy